EE Times, Junko Yoshida, January 16, 2020, https://www.eetimes.com/ces-2020-deconstructed-10-lessons/
LAS VEGAS — After miles of roaming the show floor, countless press briefings and one-on-one interviews, what did we learn at the CES 2020?
Anyone who survived the last week’s ordeal in Las Vegas almost involuntarily comes away with a personal “takeaway” list. Below are the main perceptions I derived from my tour of CES 2020.
[Separately I recorded my interviews with executives from leading automotive semiconductor companies and aired them on our radio show, CES 2020, Day 3: IC Vendors Talk Self-Driving. Please give it a listen.]
1. Camera-only AVs can create ‘internal redundancy’
Forget radars and lidars?
Experts in the automated vehicle (AV) industry maintain that redundancy — essential to the safety of AVs — comes from the use of multiple sensory modalities (i.e. vision, radar, lidar), and data fusion.
At an Intel/Mobileye press briefing at CES last week, Amnon Shashua, president and CEO of Mobileye, defied conventional wisdom. He said that by leveraging AI advancements, Mobileye now runs different neural network algorithms on multiple independent computer vision engines. This, he said, creates “internal redundancies.”
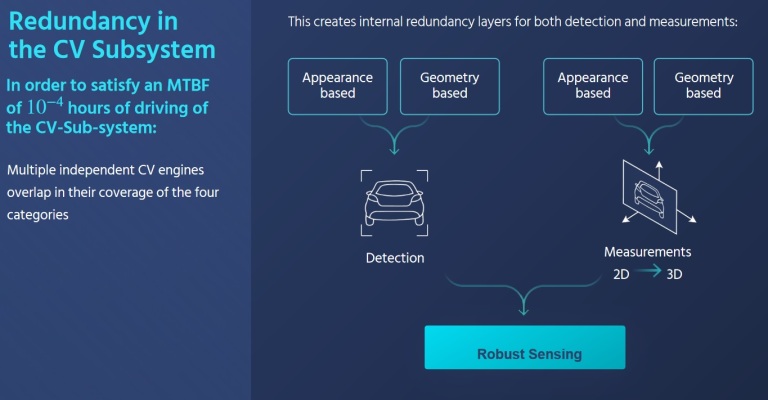
For example, Mobileye says it applies as many as six different algorithms to the camera-only subsystem for object detection. Separately, it runs four different algorithms to add depth to 2D images, which the company claims can effectively create 3D images without using lidars. Some of the new neural networks used by Mobileye include “Parallax Net” capable of providing accurate structure understanding, “Visual Lidar” (Vidar) offering DNN-based multi-view stereo, and “Range Net” for metric physical range estimation.
Mobileye, however, isn’t suggesting that the automotive industry do away with radars or lidars. OEMs can use a combination of lidars and radars, for example, as a separate stream, if so desired, says Shashua.
“It will be up to their customers,” said Phil Magney, founder and principal of VSI Labs. But what’s new here, Magney said, is, “the evolution of AI that can now add a certain level of redundancies to camera-only sub-systems.”
2. Filter ‘big data’
Big data is what drives connected devices. No question. But even more important is how to extract quality information out of big data. That’s where everyone struggles.
Take highly automated vehicles, for example. A processing unit powerful enough to digest incoming sensory data – whose volume gets bigger every day – won’t be cheap. A big CPU/GPU tends to dissipate too much heat. And sending big data to the cloud for AI training gets expensive. Add to this the mounting cost of annotating big data.
One way to buck the trend is to filter the data at the edge.
Teraki is a good example. The Berlin, Germany-based company claims it has developed software technology that can “adaptively resize and filter data, for more accurate object detection and machine learning.” Lidars generate “point clouds” — a collection of points that represent a 3D shape or feature, and they come with an enormous data load. What’s needed, said Teraki CEO Daniel Richart, is software that extracts information — “fast enough, at the quality they need” — before the data goes a central sensory data fusion unit inside an embedded system for transmission to the cloud.
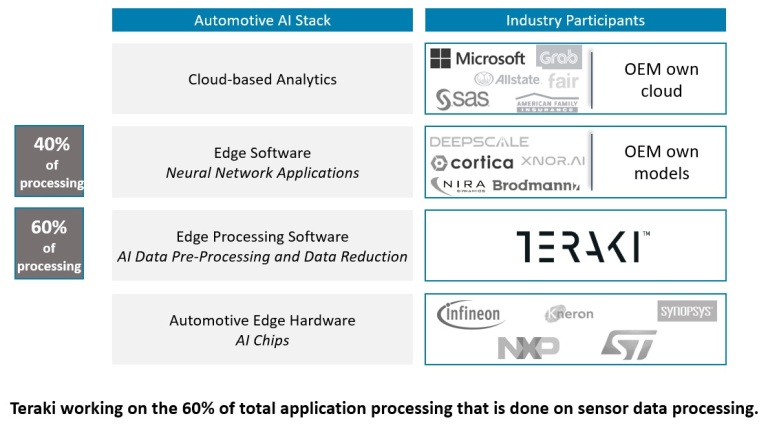
Paris, France-based Prophesee is another case. Its event-driven image sensors can directly address the issue of latency. Think of automatic emergency braking (AEB). Without waiting for an ADAS-equipped vehicle to finish fusing sensory data and issue a safety warning, the event-based camera can spot a road anomaly without latency. In comparison, frame-based images fused with radar data often miss the object on the road, because automakers do not want false positives to confuse drivers, explained Luca Verre, Prophesee CEO.
3. AI chips on 40nm to be fabricated in Japan
CES is not exactly a venue where AI chip startups set up booths for demos. Nonetheless, AI chip company executives were on the prowl. AI hardware vendors such as Blaize and Gyrfalcon were present in technical posters at their partners’ booths.
EE Times, however, caught up with Mythic co-founder and CEO Mike Henry in a press room. Mythic’s accelerators, based on a compute-in-memory approach, will be fabricated in Japan, by Mie Fujitsu Semiconductor.
With technologies that range from ultra-low-power and non-volatile memory to RF, Mie Fujitsu, now wholly owned by UMC, offers foundry services based on 300mm wafer production facilities. Mythic will shortly start sampling its first AI accelerator chip — integrated with a PCI express interface to connect to the host — together with its SDK, according to Henry.
In the AI race, on one end of the spectrum, companies such as Nvidia have pioneered a large AI model equipped with faster acceleration, lower latency and higher resolution. On the other end of the spectrum, chips are running a tiny machine-learning model, which, however, could suffer a huge hit on accuracy, Henry explained. “The industry is currently stuck.”
Mythic hopes that by splitting the difference it will occupy a sweet spot. It is developing in-memory AI acceleration chips powerful enough to handle HD video at 30 frames per second, while achieving low latency and low cost. System vendors need not pay for the memory bandwidth.
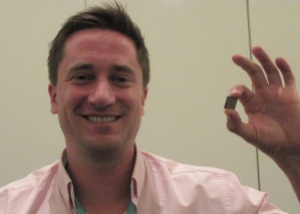
For what sort of applications will such an AI acceleration chip be used?
“This is for high quality products,” explained Henry. AI acceleration can enhance image quality, even in low light. It can even detect and read license plates. In a parking garage, it could augment security. In short, AI can take over a lot of challenging high-quality video-capturing and processing tasks that traditional cameras can’t pull off without inventing new hardware.
The key to Mythic’s compute in memory approach is a Flash memory array combined with ADCs and DACs, turned into a matrix multiply engine. On the Mythic chip Henry is holding in the picture above, 10,000 ADCs are crammed inside.
4. Selling into the future
Clearly, the initial euphoria of consumer IoT devices is over.
Most consumers think nothing of replacing smartphones or wearable devices in less than a year. In contrast, connected devices for the industrial market are a long game.
That applies equally to highly connected vehicles and Industrial IoT. As Gideon Wertheizer, CEO of CEVA, explained, many building blocks used in today’s highly automated vehicles (or industrial IoT devices) are not so different from what the industry has already developed. “The difference is whether you have the patience to stay in that [long-haul] business.”
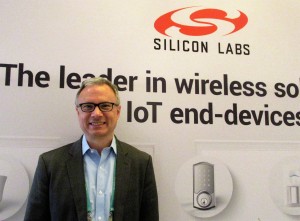
Patience is one. Commitment is another.
Silicon Labs’ CEO Tyson Tuttle told us that, as IoT continues to look for design wins in the industrial market, the chip supplier’s job is never done at time of sale. He said, “We are selling our chips into the future.” More specifically, the success of the industrial IoT business is incumbent on chip suppliers’ commitment to support new software, protocol updates and applications. “All that over the next 10 to 15 years.”
5. Let big data companies see the forest but not trees
In the era of big data, if you are interested in the protection of your private data or increasingly concerned about future AI applications, look to Europe for help.
Unlike U.S. regulatory agencies who are hesitant to challenge big tech corporations’ “innovations,” European regulators have squarely committed protecting their citizens. Privacy and AI are their top agenda items.
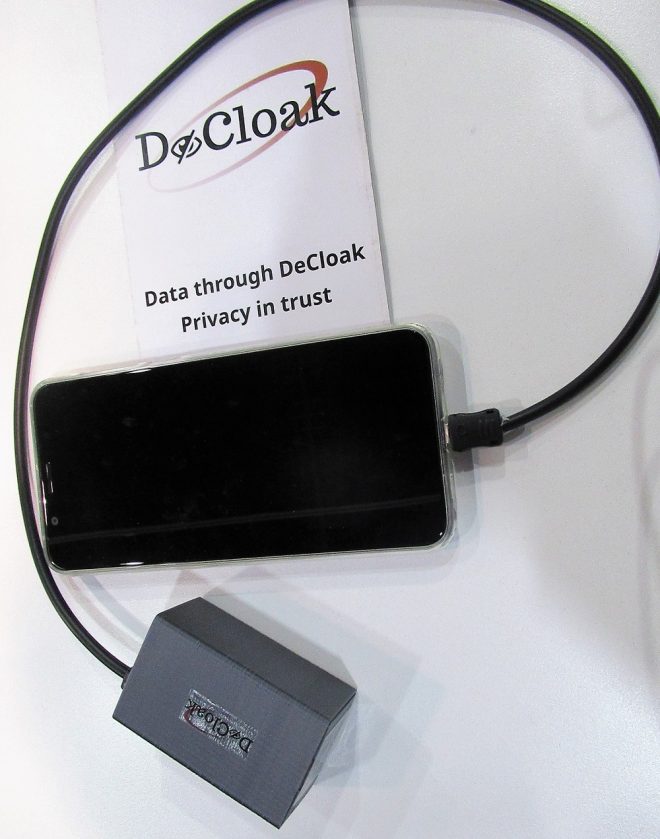
The EU’s General Data Protection Regulation (GDPR) is already setting the tone not just for Europe but for the worldwide market. Global companies engaged in aggregation and data analysis are mindful that their business practices do not violate the GDPR.
At CES last week, we came across a Taiwan-based startup called DeCloak. The company’s founders succinctly described their technology as “letting big data companies see the forest without seeing any trees.”
In the burgeoning confluence of surveillance and social media, the idea of “de-cloaking” might have never occurred to Silicon Valley startups.
DeCloak has designed a privacy processing unit (PPU) on a 1x1mm chip. The PPU includes a true random number generator. Installed in a dongle connected to a smartphone, for example, the PPU would block any private data that would otherwise migrate automatically to the cloud.
DeCloak believes that de-identification is vital for medical data. Although data collectors often say they are anonymizing data, it’s unclear how much are they doing. Using the PPU, a patient can de-identify himself, to comfortably participate in the sort of medical research that depends on big data, according to DeCloak.
Source: EE Times